Team: Quantum Computing in AI4EO
Quantum mechanics has stirred interest in a number of research fields over the last years, including data processing. This is especially due to the promise of Quantum computers (QC) to solve specific problems faster and more efficient than conventional classical algorithms. Following this direction, our team focuses on different ways to exploit subfields of quantum mechanics, such as QC and quantum machine learning (QML), for earth observation (EO) tasks and explores how they can help shape the future of large scale data processing and classification.
Fundamental research — Complexity and simulability of quantum states
As of now it has proven difficult to determine whether for a given computational problem a fast quantum algorithm exists that outperforms classical algorithms running on (super) computers. Many computational tasks, like the simulation of quantum systems, can oftentimes be efficiently executed on classical computers through cleverly designed Monte Carlo algorithms. Thus, a precise description of the boundary between quantum and classical simulability remains difficult. To shed light on this important question, part of our team studies the complexity of quantum states and their classical simulability. To be precise, we investigate different notions of complexity and their use in examples such as quantum walks. Furthermore, we study the classical simulability of quantum systems through Monte Carlo sampling techniques. The outcome of these investigations promises to help us understand the circumstances under which quantum computational resources are essential and must be deployed.

Bhilahari Jeevanesan, Phys. Rev. A 110, 032206 – Published 6 September 2024
https://journals.aps.org/pra/abstract/10.1103/PhysRevA.110.032206
Applied Theory — Quantum machine learning in EO data classification
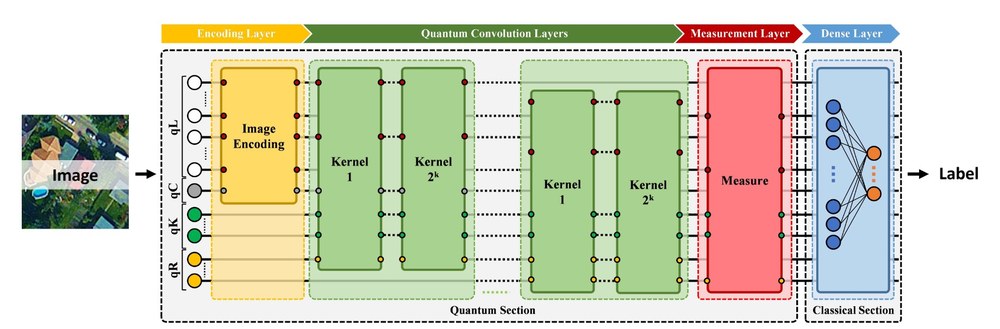
F. Fan, Y. Shi, T. Guggemos and X. X. Zhu, "Hybrid Quantum-Classical Convolutional Neural Network Model for Image Classification," in IEEE Transactions on Neural Networks and Learning Systems, doi: 10.1109/TNNLS.2023.3312170.
https://ieeexplore.ieee.org/abstract/document/10254235
Another challenge with respect to QC is the fact that today's functional prototypes only consist of a very limited number of quantum bits, which restricts the size of the input information. Thus the second focus of our team is to determine if and how these 'small' quantum computers can be used to enhance EO data processing and classification. To be precise, we focus on QML algorithms, which combine quantum computing with machine learning, to tackle this task.
In particular, we explore two different strategies:
1. Use classical preprocessing to extract a small number of features and subsequently employ QML to classify the image based on these features
2. Use QML to preprocess the image to extract relevant features and a classical convolutional neural networt (CNN) for classification. This approach is called Quantum Classical Convolutional Neural Network (QC-CNN)
1. QML for classification
This strategy is investigated for different QML platforms such as quantum annealers and gate based quantum computers. The efficiency of this approach can then be tested on various test data sets and so far usually seems to be on par with its classical counterparts, even outperforming it in certain circumstances. For further enhancing we are researching in decreasing or even circumventing the required amount of classical preprocessing.
2. QC-CNN
The combination of feature extraction with QML and subsequent classification with a classical CNN was first successfully demonstrated for radically downscaled (8 by 8 bit pixels) grey-scale EO images. Since then our focus has shifted on how to encode larger multi-spectral images using enhanced ‘superpixel’ preprocessing to avoid lossy downscaling and different techniques such as MCQI and FRQI to encode and process multiple spectral bands at the same time. We have since been able to implement both improvements and demonstrate their advantages compared to both classical and other quantum schemes. However, we are determined to try to find new ways to increase the efficiency and functionality of our scheme.
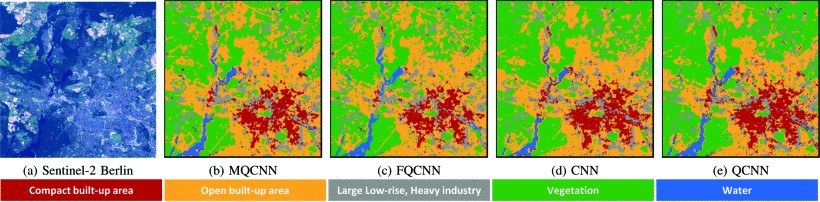
F. Fan, Y. Shi and X. X. Zhu, "Urban Land Cover Classification from Sentinel-2 Images with Quantum-Classical Network," 2023 Joint Urban Remote Sensing Event (JURSE), Heraklion, Greece, 2023, pp. 1-4, doi: 10.1109/JURSE57346.2023.10144213.
https://ieeexplore.ieee.org/document/10144213
Hybrid HPC-QC
We are part of a European team dedicated to combine High Performance Computers (HPC) with Quantum Computers, to make QC relevant and accessible for other research fields. This combination of HPC and QC could prove useful for a number of applications such as energy anomaly detection, vegetation management, transport route optimization and image classification. While our focus regarding this project will be on the last topic, we believe that we will be able to contribute largely to the general idea and implementation of this promising crossover of high level classical and quantum computing.
In collaboration with the DLR_School_Labs, we plan to use this research as an outreach platform for schools and the general public. We believe that the study of earth sciences is a great motivation to learn about the concepts of quantum mechanics and quantum computing.
Experiment — QML on photonic chips
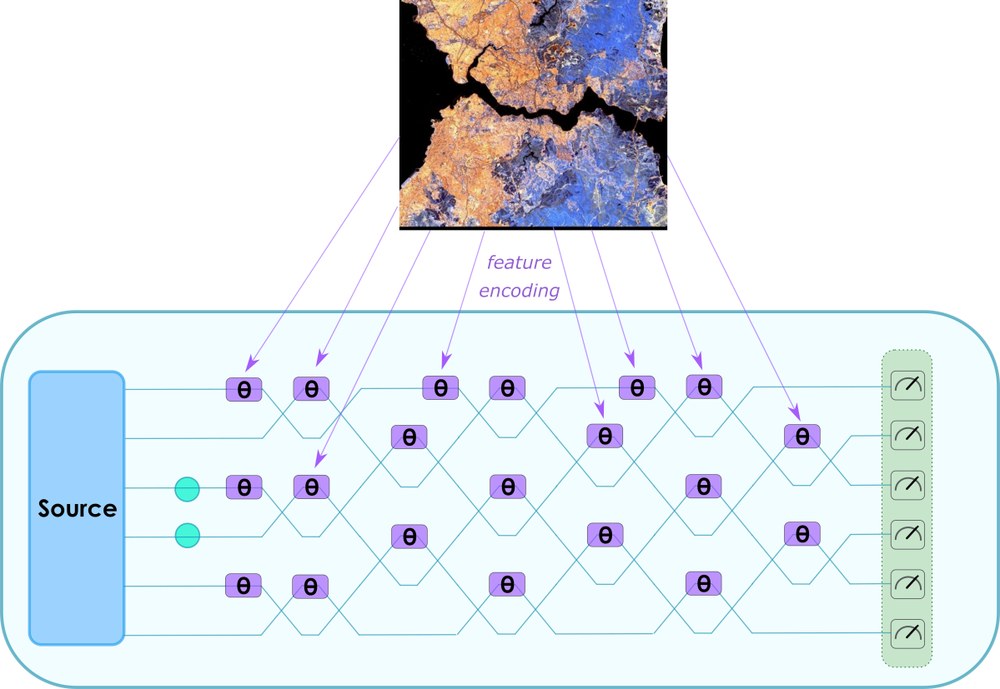
The last pillar of our research is the actual experimental implementation of QML. In a collaboration together with the University of Vienna we aim to implement QML algorithms for EO data processing and classification on a photonic chip. To this end we consider Quantum Kernel Methods, Quantum Neural Networks and Quantum Reservoir Computing as promising candidates. The final goal is thus to not only test different preexisting universal quantum hardware for EO tasks but to also design and fabricate a photonic chip specifically for our precise applications.