Quantum computing project Klim-QML starts with companies d-fine and planqc
Klim-QML is a project of the DLR Institute of Atmospheric Physics (IPA) within the DLR Quantum Computing Initiative (DLR QCI), which aims to exploit the potential of quantum computing in improving climate models and to develop the first prototype of a climate model improved with quantum machine learning techniques. This should reduce uncertainties in climate predictions for robust technology impact assessments and enable mitigation recommendations for diverse applications in aerospace, transport and energy. The project is funded by the German Federal Ministry for Economic Affairs and Climate Action and builds on extensive experience gained at the IPA under the ERC Synergy Grant USMILE in the area of improving climate models with machine learning.
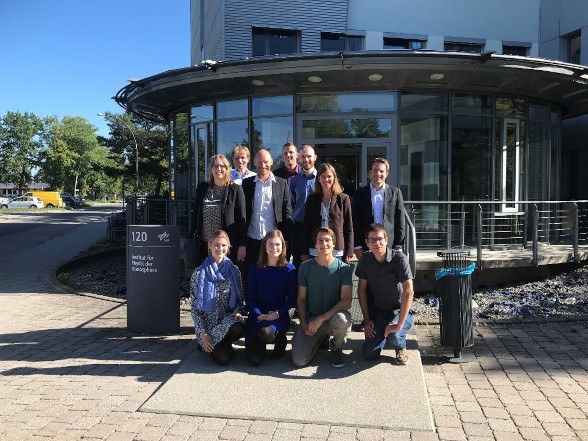
Now Klim-QML has found contractors to support the development and implementation of quantum computing algorithms: A consortium of the consultancy d-fine and the Garching-based neutral atom start-up planqc will support the DLR team led by Dr Mierk Schwabe and Prof Veronika Eyring in unlocking the computational potential of DLR's QCI quantum computers for applications in climate research. Planqc is already involved in the DLR QCI with the DiNAQC project. The kick-off was celebrated with these companies on 21 September.
Earth System Models (ESMs) simulate the physical climate and biogeochemical cycles under a variety of influences and are essential in predicting the changing climate. However, there are persistent uncertainties in their predictions, mainly caused by the representation of processes that occur on scales smaller than the resolution of the model grid. These processes must be approximated by parameterisations.
Simulations with cloud-resolving models are extremely computationally intensive and can therefore only be used for a very short period of time or only for a small region. Relying solely on supercomputing performance improvements is therefore not a solution. Within USMILE, cloud-resolving models are used to train machine learning algorithms, which can eliminate systematic errors in climate models and contribute to a significant speed-up of the models.

Within the framework of Klim-QML, the existing expertise will be built upon and the work will be extended to quantum machine learning, whose methods are potentially better compared to classical approaches, e.g. can be trained better and generalise better to data not used in training. In addition, first methods are being developed to use quantum computers in setting free parameters of climate models. D-fine and planqc will be responsible for the concrete implementation on DLR QCI quantum computers. Finally, the climate model prototype will be employed with a use case and evaluated in detail with the help of observational data using the ESMValTool developed at IPA.
"We see great potential to further improve and accelerate the models using quantum computing," says Veronika Eyring. Mierk Schwabe adds: "By researching this essential topic, we are building important competencies for the future."