Shake-The-Box: 3D Lagrangian Particle Tracking at high Particle Densities
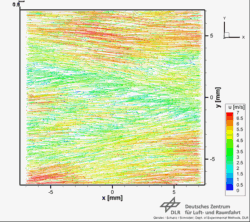
Shake-The-Box (STB) is a novel time-resolved 3D Lagrangian particle tracking method for measuring densely seeded flows. The STB algorithm has been developed at the department of Experimental Methods at DLR Göttingen in the past few years and uses the prediction of 3D particle distributions for each subsequent time-step as a mean to seize the temporal domain within a time series for accurate track reconstructions. Due to the heavy incorporation of temporal data, STB can also be termed 4D-PTV (Particle Tracking Velocimetry).
The particle distribution is closely sampled by few (typically three to five) cameras at different viewing angles. Before performing the real measurements, a 3D camera calibration is performed, followed by a volume-self-calibration and the determination of the local Optical Transfer Function (OTF). STB overcomes the ill-posed reconstruction problem for 3D particle distributions at high seeding densities present for each single time-step (as known for tomo-PIV and classical PTV) by pre-solving the problem for each predicted time-step using an extrapolation strategy. To this end, a temporal filtering is applied to already known trajectories from previous time-steps and extrapolated to the current time-step. This central step is followed by a ‘shake’-process, which corrects the involved prediction error by an accurate image-matching scheme (a method that is also applied within the Iterative Particle Reconstruction technique).
Exploiting the temporal information enables the processing of particle images of densely seeded flows (up to and beyond 0.1 particles per pixel). Such particle image densities exceed ~10 times the limits of classical PTV algorithms. Furthermore, the computing time for a STB reconstruction is about 5 to 20 times faster than the fastest actual Tomo-PIV algorithm (depending on particle number and volume depth).

With STB, full trajectories of particles - which at low Stokes numbers can be considered as fluid elements - can be identified at high spatial accuracy due to a nearly complete suppression of ghost particles. A temporal filtering scheme (optimal weighted 1D B-Spline) further improves on accuracy and allows for the extraction of local 3C velocity and acceleration as derivatives of a continuous function with a high dynamic range.
Given such dense velocity information at irregularly distributed 3D positions of fluid elements in a (turbulent) flow enables the application of a velocity and acceleration interpolation scheme onto an Eulerian (regular) grid. Such an interpolation algorithm, termed FlowFit, has been developed in parallel to STB at our department. It has been found to be superior to classical low-pass filtering 3D cross-correlation methods, especially when applying regularization by Navier-Stokes constraints.
To reconstruct the velocity field as a continuous function based on scattered particle data, we model this function as a uniform cubic B-spline and compute the weighting coefficients of the B-spline's compact base functions by minimizing a cost function. This cost function is the sum of different kinds of squared and weighted errors including the errors between reconstructed velocity and measured velocity at the particle locations. As regularization, the curvature at each grid point is part of the cost function with a selectable but typically low weight. This results in an optimization problem with a unique solution. Additional regularizations are possible: in case of an incompressible flow we can effectively penalize the divergence of the solutions in the cost function. The resulting flow field is then sampled on a regular grid including its spatial derivatives so that the derived values, such as vorticity or Q-criterion, can be computed without numerical differentiation.
Thus, a subsequent evaluation of a time series of particle images from few projections with STB and FlowFit delivers the full volumetric Lagrangian velocity and acceleration information on single fluid elements along trajectories (output of STB) together with the full time-resolved velocity- and acceleration- gradient tensor on an Eulerian regular grid (output of FlowFit). The former enables a bin averaging process, which can deliver mean and corresponding Reynolds stress profiles with sub-pixel spatial resolution.