shepard - storage for heterogeneous product and research data
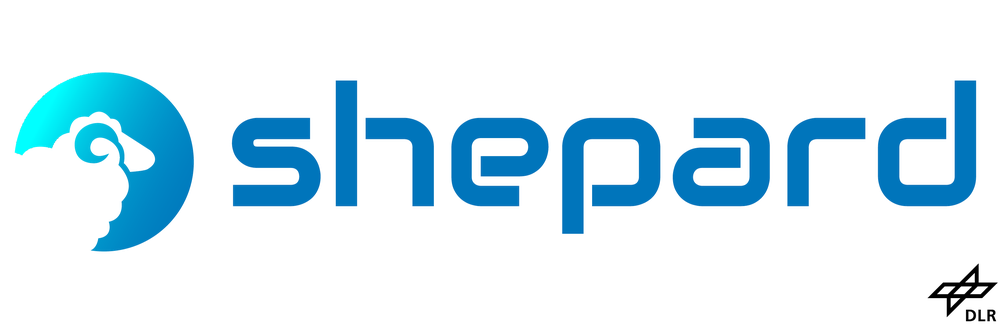
DLR
DLR
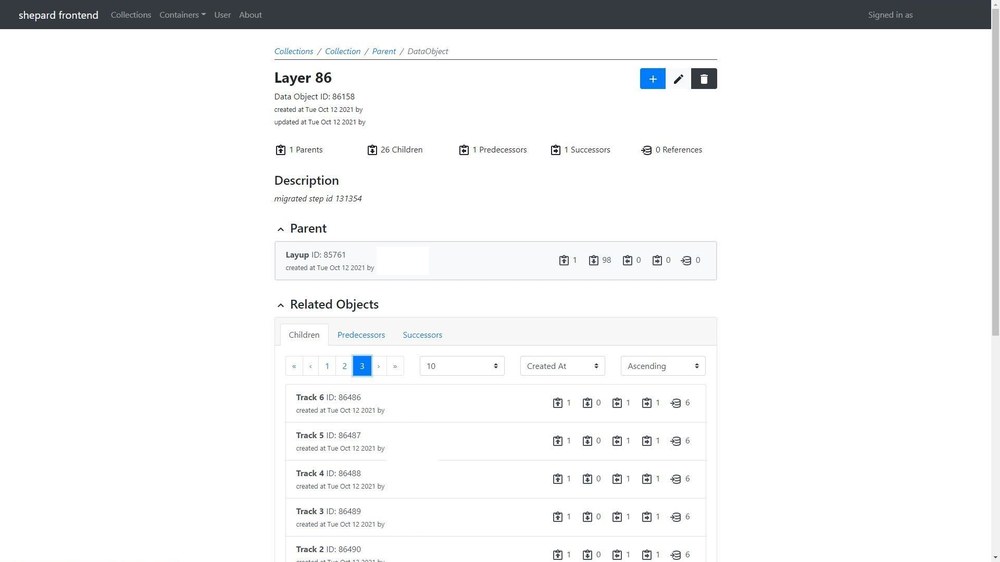
DLR
Guided by the vision of seamless digital integration of a wide variety of process chains, the shepard system (storage for heterogeneous product and research data) is being developed at the Centre for Lightweight Production Technology in Augsburg. One focus here is the cross-disciplinary utilisation of all generated data for AI methods for data analysis or contextualised data curation, among other things.
Shepard is a scalable system for the highly flexible automated storage and linking of heterogeneous data (e.g. measured values, simulation results, CAD data) and metadata (e.g. provenance, semantic categorisation) along a wide variety of real and digital process chains. It is intended to offer all employees a simple and sustainable way to store, retrieve, analyse and share research data for cross-disciplinary collaboration and thus forms the basis for end-to-end research data management from experiment to publication.
Through the development and prototypical use for the structured recording of experiments in a wide variety of disciplines (from virtual simulation workflows and production technology to flight experiments or a laser free beam path), the system can already cover many domains in the context of research, especially DLR's research fields, and simple connection options via standardised interfaces enable the automated recording of data including annotation with meta-information. These interfaces are also used for analysis and form the basis for connecting any AI framework. Shepard's basic functions can be used conveniently via the web interface. More complex applications can be connected via the REST API provided.
The basic architecture of shepard includes the linking of different existing databases for the optimised storage and linking of highly heterogeneous data sets. The consistent use of open source technologies avoids vendor lock-in and enables the system to be operated free of charge. Nevertheless, many components also have corresponding enterprise licensing models to guarantee long-term scalability.
In future, the existing functions will be expanded to include more complex search queries via content, improved visualisations and the connection of internal and external tools. Shepard was published using the Apache 2.0 licence on Gitlab at https://gitlab.com/dlr-shepard. The active participation of external interested parties or contributors is expressly welcomed.
This approach opens up a broad community for detailed feedback and continuous further development and at the same time contributes to the digital transformation of science.